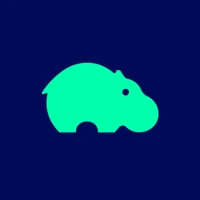
image
imagewidth (px) 10
658
| item_ID
stringlengths 13
13
| query
stringclasses 1
value | title
stringlengths 1
711
⌀ | position
int64 0
0
|
---|---|---|---|---|
id-0000000116 | None | A Logbook for Your Life - 30 Days to Change: Stress: The Intelligent Trucker Changes Series | 0 |
|
id-0000044806 | None | Medimix Ayurvedic Facewash - 100 ml (Pack of 2) | 0 |
|
id-0000143499 | None | Marvelous Meals (Good Eats Vol. 6) | 0 |
|
id-0000401048 | None | The rogue of publishers' row;: Confessions of a publisher (A Banner Book) | 0 |
|
id-000065650X | None | Everyuth Orange Peel Off - Home Facial 50 Grams (Pack of 2) | 0 |
|
id-0000659045 | None | Central Service Leadership Manual | 0 |
|
id-000077135X | None | Bloomberg Businessweek Magazine (April 1, 2013 - April 7, 2013) Samsung's Secret | 0 |
|
id-0000791156 | None | Spirit Led—Moving By Grace In The Holy Spirit's Gifts | 0 |
|
id-0001001787 | None | Sailing | 0 |
|
id-0001006657 | None | I Want My Dinner | 0 |
|
id-000102521X | None | The Fatal Strand (Tales from the Wyrd Museum) | 0 |
|
id-0001035649 | None | Under Milk Wood Dylan Thomas & the Original Cast | 0 |
|
id-0001048252 | None | All the Pretty Horses | 0 |
|
id-0001127748 | None | Lift Him up - Volume 1 | 0 |
|
id-0001237756 | None | The New Year's Imp | 0 |
|
id-0001381512 | None | Little Richard and Prickles | 0 |
|
id-0001601547 | None | Mystery of the Fiery Eye | 0 |
|
id-0001632108 | None | Pixie Tales | 0 |
|
id-0001711237 | None | Little Black Goes to the Circus! | 0 |
|
id-0001711342 | None | Doctor Dolittle and the Pirates | 0 |
|
id-000171337X | None | Oh Say Can You Say? | 0 |
|
id-0001837397 | None | Autumn Story: Introduce children to the seasons in the gorgeously illustrated classics of Brambly Hedge! | 0 |
|
id-000184086X | None | The High Hills (Brambly Hedge) | 0 |
|
id-0001846590 | None | Princess and the Goblin (Abridged Classics) | 0 |
|
id-0001939777 | None | The Lion, the Witch and the Wardrobe (The Chronicles of Narnia) | 0 |
|
id-0001942263 | None | Little Grey Rabbit's May Day (Little Grey Rabbit Library) | 0 |
|
id-0001955071 | None | Mog the Forgetful Cat | 0 |
|
id-0001961853 | None | Sinister Wisdom 41 Summer/Fall 1990 | 0 |
|
id-0001983415 | None | Nice for Mice | 0 |
|
id-0001983458 | None | A Visit to Brambly Hedge | 0 |
|
id-0001983660 | None | Bloomer | 0 |
|
id-0002000423 | None | Fumbling with a Flyrod : Stories of the River | 0 |
|
id-0002005573 | None | Bedlam | 0 |
|
id-0002005786 | None | Garden of Venus | 0 |
|
id-0002006804 | None | Life on the Refrigerator Door | 0 |
|
id-0002007185 | None | Woolf in Ceylon : An Imperial Journey in the Shadow of Leonard Woolf, 1904-1911 | 0 |
|
id-0002007533 | None | Donna Hay Christmas: Simple Recipes, Menu Planners | 0 |
|
id-0002008068 | None | Lullabies for Little Criminals unknown Edition by O'Neill, Heather [2006] | 0 |
|
id-0002008483 | None | Curl to Win | 0 |
|
id-0002111616 | None | De Gaulle: A biography | 0 |
|
id-0002111640 | None | Farewell to the Don: The journal of Brigadier H. N. H. Williamson; | 0 |
|
id-0002114836 | None | The lure of the falcon | 0 |
|
id-0002116324 | None | MARGO OLIVER'S WEEKEND MAGAZINE COOK BOOK | 0 |
|
id-0002117088 | None | Renoir, My Father | 0 |
|
id-0002117908 | None | Social Contract: A Personal Inquiry into the Evolutionary Sources of Order and Disorder | 0 |
|
id-0002153572 | None | Wild swans: Three daughters of China | 0 |
|
id-000215479X | None | Practicalities | 0 |
|
id-0002154803 | None | Letters: C.S. Lewis & Don Giovanni Calabria | 0 |
|
id-000215854X | None | America: The Beautiful Cookbook | 0 |
|
id-0002159309 | None | Shining Path: The world's deadliest revolutionary force | 0 |
|
id-000216194X | None | The life of my choice | 0 |
|
id-0002163322 | None | THE SALAD DAYS: AN AUTOBIOGRAPHY [LARGE PRINT] | 0 |
|
id-000216373X | None | Ashkenazy: Beyond Frontiers | 0 |
|
id-0002173689 | None | A Field Guide to the Nests, Eggs and Nestlings of North American Birds (Collins Pocket Guide) | 0 |
|
id-0002177137 | None | The Lion and the Honeycomb | 0 |
|
id-0002178273 | None | Jaguar One Man's Struggle to Save Jaguars in the Wild | 0 |
|
id-0002189364 | None | Michael Owen Soccer Skills (Collins GEM) | 0 |
|
id-0002190044 | None | Pyramids of life: An investigation of nature's fearful symmetry | 0 |
|
id-0002191911 | None | Birds of the West Indies | 0 |
|
id-000219502X | None | People of the Lake: Man, His Origins, Nature, and Future | 0 |
|
id-0002200775 | None | Birds of Southern South America and Antarctica (Collins Illustrated Checklist) | 0 |
|
id-0002202085 | None | London 360: Views Inspired by British Airways London Eye | 0 |
|
id-0002214873 | None | The lost embassy | 0 |
|
id-0002215470 | None | Breakheart Pass | 0 |
|
id-0002216949 | None | Ransom | 0 |
|
id-0002218577 | None | The Unconquerable | 0 |
|
id-0002226049 | None | The Last Frontier | 0 |
|
id-0002226723 | None | North and South | 0 |
|
id-0002227096 | None | The Final Run | 0 |
|
id-0002227649 | None | In honour bound | 0 |
|
id-0002228645 | None | The loving cup: A novel of Cornwall, 1813-1815 | 0 |
|
id-0002229277 | None | The Hunt for Red October | 0 |
|
id-0002231247 | None | Gray Eagles | 0 |
|
id-0002231271 | None | I Was a 15 Year Old Blimp | 0 |
|
id-0002233916 | None | Dust in Sunlight | 0 |
|
id-0002242052 | None | Without Remorse | 0 |
|
id-0002251892 | None | Muhammad Ali in Perspective | 0 |
|
id-0002251981 | None | See You Later, Litigator! (Peanuts at Work and Play) | 0 |
|
id-0002252104 | None | Grains (Gourmet Pantry) | 0 |
|
id-0002254409 | None | Seahorses | 0 |
|
id-0002256045 | None | The Willows at Christmas | 0 |
|
id-0002261820 | None | One for my baby | 0 |
|
id-0002315637 | None | Nemesis | 0 |
|
id-0002315858 | None | Long Hard Cure | 0 |
|
id-0002322420 | None | Coffin in the Black Museum | 0 |
|
id-000232265X | None | The Mamur Zapt and the Donkey-Vous | 0 |
|
id-0002325128 | None | Asking for the Moon | 0 |
|
id-0002326809 | None | When the Ashes Burn | 0 |
|
id-0002435039 | None | Mrs Tim | 0 |
|
id-000250653X | None | Jesus of Nazareth | 0 |
|
id-0002551446 | None | Shaka's children: A history of the Zulu people | 0 |
|
id-0002551489 | None | The Best of Mexico | 0 |
|
id-0002551519 | None | Pacific Northwest: The Beautiful Cookbook | 0 |
|
id-0002551659 | None | Lemons: A Country Garden Cookbook | 0 |
|
id-000255206X | None | The Best of Thailand: A Cookbook | 0 |
|
id-000255349X | None | Halliwell's Film Guide | 0 |
|
id-0002553708 | None | Mediterranean the Beautiful Cookbook: Authentic Recipes from the Mediterranean Lands | 0 |
|
id-0002553899 | None | Rare Air: Michael on Michael | 0 |
|
id-0002554216 | None | A Heart for Children: Inspirations for Parents and Their Children | 0 |
|
id-0002556642 | None | Stanley Spencer: A Biography | 0 |
Marqo Ecommerce Embedding Models
In this work, we introduce the AmazonProducts-3m dataset for evaluation. This dataset comes with the release of our state-of-the-art embedding models for ecommerce products: Marqo-Ecommerce-B and Marqo-Ecommerce-L.
Released Content:
- Marqo-Ecommerce-B and Marqo-Ecommerce-L embedding models
- GoogleShopping-1m and AmazonProducts-3m for evaluation
- Evaluation Code
The benchmarking results show that the Marqo-Ecommerce models consistently outperformed all other models across various metrics. Specifically, marqo-ecommerce-L
achieved an average improvement of 17.6% in MRR and 20.5% in nDCG@10 when compared with the current best open source model, ViT-SO400M-14-SigLIP
across all three tasks in the marqo-ecommerce-hard
dataset. When compared with the best private model, Amazon-Titan-Multimodal
, we saw an average improvement of 38.9% in MRR and 45.1% in nDCG@10 across all three tasks, and 35.9% in Recall across the Text-to-Image tasks in the marqo-ecommerce-hard
dataset.
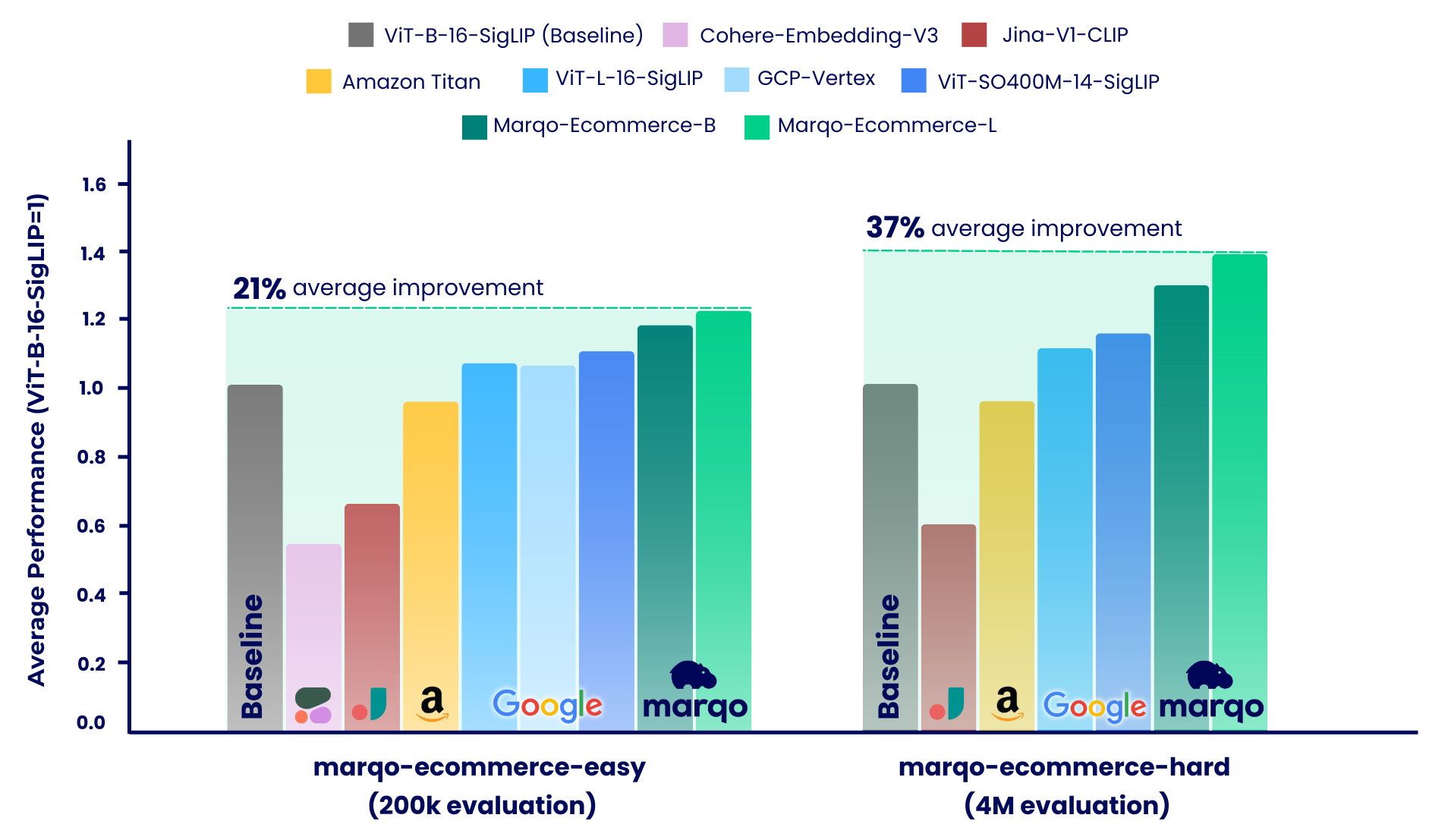
More benchmarking results can be found below.
Models
Embedding Model | #Params (m) | Dimension | HuggingFace | Download .pt |
---|---|---|---|---|
Marqo-Ecommerce-B | 203 | 768 | Marqo/marqo-ecommerce-embeddings-B | link |
Marqo-Ecommerce-L | 652 | 1024 | Marqo/marqo-ecommerce-embeddings-L | link |
Load from HuggingFace with transformers
To load the models in Transformers, see below. The models are hosted on Hugging Face and loaded using Transformers.
from transformers import AutoModel, AutoProcessor
import torch
from PIL import Image
import requests
model_name= 'Marqo/marqo-ecommerce-embeddings-L'
# model_name = 'Marqo/marqo-ecommerce-embeddings-B'
model = AutoModel.from_pretrained(model_name, trust_remote_code=True)
processor = AutoProcessor.from_pretrained(model_name, trust_remote_code=True)
img = Image.open(requests.get('https://raw.githubusercontent.com/marqo-ai/marqo-ecommerce-embeddings/refs/heads/main/images/dining-chairs.png', stream=True).raw).convert("RGB")
image = [img]
text = ["dining chairs", "a laptop", "toothbrushes"]
processed = processor(text=text, images=image, padding='max_length', return_tensors="pt")
processor.image_processor.do_rescale = False
with torch.no_grad():
image_features = model.get_image_features(processed['pixel_values'], normalize=True)
text_features = model.get_text_features(processed['input_ids'], normalize=True)
text_probs = (100 * image_features @ text_features.T).softmax(dim=-1)
print(text_probs)
# [1.0000e+00, 8.3131e-12, 5.2173e-12]
Load from HuggingFace with OpenCLIP
To load the models in OpenCLIP, see below. The models are hosted on Hugging Face and loaded using OpenCLIP. You can also find this code inside run_models.py
.
pip install open_clip_torch
from PIL import Image
import open_clip
import requests
import torch
# Specify model from Hugging Face Hub
model_name = 'hf-hub:Marqo/marqo-ecommerce-embeddings-L'
# model_name = 'hf-hub:Marqo/marqo-ecommerce-embeddings-B'
model, preprocess_train, preprocess_val = open_clip.create_model_and_transforms(model_name)
tokenizer = open_clip.get_tokenizer(model_name)
# Preprocess the image and tokenize text inputs
# Load an example image from a URL
img = Image.open(requests.get('https://raw.githubusercontent.com/marqo-ai/marqo-ecommerce-embeddings/refs/heads/main/images/dining-chairs.png', stream=True).raw)
image = preprocess_val(img).unsqueeze(0)
text = tokenizer(["dining chairs", "a laptop", "toothbrushes"])
# Perform inference
with torch.no_grad(), torch.cuda.amp.autocast():
image_features = model.encode_image(image, normalize=True)
text_features = model.encode_text(text, normalize=True)
# Calculate similarity probabilities
text_probs = (100.0 * image_features @ text_features.T).softmax(dim=-1)
# Display the label probabilities
print("Label probs:", text_probs)
# [1.0000e+00, 8.3131e-12, 5.2173e-12]
Evaluation
Generalised Contrastiove Learning (GCL) is used for the evaluation. The following code can also be found in scripts
.
git clone https://github.com/marqo-ai/GCL
Install the packages required by GCL.
1. GoogleShopping-Text2Image Retrieval.
cd ./GCL
MODEL=hf-hub:Marqo/marqo-ecommerce-B
outdir=/MarqoModels/GE/marqo-ecommerce-B/gs-title2image
hfdataset=Marqo/google-shopping-general-eval
python evals/eval_hf_datasets_v1.py \
--model_name $MODEL \
--hf-dataset $hfdataset \
--output-dir $outdir \
--batch-size 1024 \
--num_workers 8 \
--left-key "['title']" \
--right-key "['image']" \
--img-or-txt "[['txt'], ['img']]" \
--left-weight "[1]" \
--right-weight "[1]" \
--run-queries-cpu \
--top-q 4000 \
--doc-id-key item_ID \
--context-length "[[64], [0]]"
2. GoogleShopping-Category2Image Retrieval.
cd ./GCL
MODEL=hf-hub:Marqo/marqo-ecommerce-B
outdir=/MarqoModels/GE/marqo-ecommerce-B/gs-cat2image
hfdataset=Marqo/google-shopping-general-eval
python evals/eval_hf_datasets_v1.py \
--model_name $MODEL \
--hf-dataset $hfdataset \
--output-dir $outdir \
--batch-size 1024 \
--num_workers 8 \
--left-key "['query']" \
--right-key "['image']" \
--img-or-txt "[['txt'], ['img']]" \
--left-weight "[1]" \
--right-weight "[1]" \
--run-queries-cpu \
--top-q 4000 \
--doc-id-key item_ID \
--context-length "[[64], [0]]"
3. AmazonProducts-Category2Image Retrieval.
cd ./GCL
MODEL=hf-hub:Marqo/marqo-ecommerce-B
outdir=/MarqoModels/GE/marqo-ecommerce-B/ap-title2image
hfdataset=Marqo/amazon-products-eval
python evals/eval_hf_datasets_v1.py \
--model_name $MODEL \
--hf-dataset $hfdataset \
--output-dir $outdir \
--batch-size 1024 \
--num_workers 8 \
--left-key "['title']" \
--right-key "['image']" \
--img-or-txt "[['txt'], ['img']]" \
--left-weight "[1]" \
--right-weight "[1]" \
--run-queries-cpu \
--top-q 4000 \
--doc-id-key item_ID \
--context-length "[[64], [0]]"
Detailed Performance
Our benchmarking process was divided into two distinct regimes, each using different datasets of ecommerce product listings: marqo-ecommerce-hard and marqo-ecommerce-easy. Both datasets contained product images and text and only differed in size. The "easy" dataset is approximately 10-30 times smaller (200k vs 4M products), and designed to accommodate rate-limited models, specifically Cohere-Embeddings-v3 and GCP-Vertex (with limits of 0.66 rps and 2 rps respectively). The "hard" dataset represents the true challenge, since it contains four million ecommerce product listings and is more representative of real-world ecommerce search scenarios.
Within both these scenarios, the models were benchmarked against three different tasks:
- Google Shopping Text-to-Image
- Google Shopping Category-to-Image
- Amazon Products Text-to-Image
Marqo-Ecommerce-Hard
Marqo-Ecommerce-Hard looks into the comprehensive evaluation conducted using the full 4 million dataset, highlighting the robust performance of our models in a real-world context.
GoogleShopping-Text2Image Retrieval.
Embedding Model | mAP | R@10 | MRR | nDCG@10 |
---|---|---|---|---|
Marqo-Ecommerce-L | 0.682 | 0.878 | 0.683 | 0.726 |
Marqo-Ecommerce-B | 0.623 | 0.832 | 0.624 | 0.668 |
ViT-SO400M-14-SigLip | 0.573 | 0.763 | 0.574 | 0.613 |
ViT-L-16-SigLip | 0.540 | 0.722 | 0.540 | 0.577 |
ViT-B-16-SigLip | 0.476 | 0.660 | 0.477 | 0.513 |
Amazon-Titan-MultiModal | 0.475 | 0.648 | 0.475 | 0.509 |
Jina-V1-CLIP | 0.285 | 0.402 | 0.285 | 0.306 |
GoogleShopping-Category2Image Retrieval.
Embedding Model | mAP | P@10 | MRR | nDCG@10 |
---|---|---|---|---|
Marqo-Ecommerce-L | 0.463 | 0.652 | 0.822 | 0.666 |
Marqo-Ecommerce-B | 0.423 | 0.629 | 0.810 | 0.644 |
ViT-SO400M-14-SigLip | 0.352 | 0.516 | 0.707 | 0.529 |
ViT-L-16-SigLip | 0.324 | 0.497 | 0.687 | 0.509 |
ViT-B-16-SigLip | 0.277 | 0.458 | 0.660 | 0.473 |
Amazon-Titan-MultiModal | 0.246 | 0.429 | 0.642 | 0.446 |
Jina-V1-CLIP | 0.123 | 0.275 | 0.504 | 0.294 |
AmazonProducts-Text2Image Retrieval.
Embedding Model | mAP | R@10 | MRR | nDCG@10 |
---|---|---|---|---|
Marqo-Ecommerce-L | 0.658 | 0.854 | 0.663 | 0.703 |
Marqo-Ecommerce-B | 0.592 | 0.795 | 0.597 | 0.637 |
ViT-SO400M-14-SigLip | 0.560 | 0.742 | 0.564 | 0.599 |
ViT-L-16-SigLip | 0.544 | 0.715 | 0.548 | 0.580 |
ViT-B-16-SigLip | 0.480 | 0.650 | 0.484 | 0.515 |
Amazon-Titan-MultiModal | 0.456 | 0.627 | 0.457 | 0.491 |
Jina-V1-CLIP | 0.265 | 0.378 | 0.266 | 0.285 |
Marqo-Ecommerce-Easy
This dataset is about 10-30 times smaller than the Marqo-Ecommerce-Hard, and designed to accommodate rate-limited models, specifically Cohere-Embeddings-v3 and GCP-Vertex.
GoogleShopping-Text2Image Retrieval.
Embedding Model | mAP | R@10 | MRR | nDCG@10 |
---|---|---|---|---|
Marqo-Ecommerce-L | 0.879 | 0.971 | 0.879 | 0.901 |
Marqo-Ecommerce-B | 0.842 | 0.961 | 0.842 | 0.871 |
ViT-SO400M-14-SigLip | 0.792 | 0.935 | 0.792 | 0.825 |
GCP-Vertex | 0.740 | 0.910 | 0.740 | 0.779 |
ViT-L-16-SigLip | 0.754 | 0.907 | 0.754 | 0.789 |
ViT-B-16-SigLip | 0.701 | 0.870 | 0.701 | 0.739 |
Amazon-Titan-MultiModal | 0.694 | 0.868 | 0.693 | 0.733 |
Jina-V1-CLIP | 0.480 | 0.638 | 0.480 | 0.511 |
Cohere-embedding-v3 | 0.358 | 0.515 | 0.358 | 0.389 |
GoogleShopping-Category2Image Retrieval.
Embedding Model | mAP | P@10 | MRR | nDCG@10 |
---|---|---|---|---|
Marqo-Ecommerce-L | 0.515 | 0.358 | 0.764 | 0.590 |
Marqo-Ecommerce-B | 0.479 | 0.336 | 0.744 | 0.558 |
ViT-SO400M-14-SigLip | 0.423 | 0.302 | 0.644 | 0.487 |
GCP-Vertex | 0.417 | 0.298 | 0.636 | 0.481 |
ViT-L-16-SigLip | 0.392 | 0.281 | 0.627 | 0.458 |
ViT-B-16-SigLip | 0.347 | 0.252 | 0.594 | 0.414 |
Amazon-Titan-MultiModal | 0.308 | 0.231 | 0.558 | 0.377 |
Jina-V1-CLIP | 0.175 | 0.122 | 0.369 | 0.229 |
Cohere-embedding-v3 | 0.136 | 0.110 | 0.315 | 0.178 |
AmazonProducts-Text2Image Retrieval.
Embedding Model | mAP | R@10 | MRR | nDCG@10 |
---|---|---|---|---|
Marqo-Ecommerce-L | 0.92 | 0.978 | 0.928 | 0.940 |
Marqo-Ecommerce-B | 0.897 | 0.967 | 0.897 | 0.914 |
ViT-SO400M-14-SigLip | 0.860 | 0.954 | 0.860 | 0.882 |
ViT-L-16-SigLip | 0.842 | 0.940 | 0.842 | 0.865 |
GCP-Vertex | 0.808 | 0.933 | 0.808 | 0.837 |
ViT-B-16-SigLip | 0.797 | 0.917 | 0.797 | 0.825 |
Amazon-Titan-MultiModal | 0.762 | 0.889 | 0.763 | 0.791 |
Jina-V1-CLIP | 0.530 | 0.699 | 0.530 | 0.565 |
Cohere-embedding-v3 | 0.433 | 0.597 | 0.433 | 0.465 |
Citation
@software{zhu2024marqoecommembed_2024,
author = {Tianyu Zhu and and Jesse Clark},
month = oct,
title = {{Marqo Ecommerce Embeddings - Foundation Model for Product Embeddings}},
url = {https://github.com/marqo-ai/marqo-ecommerce-embeddings/},
version = {1.0.0},
year = {2024}
}
- Downloads last month
- 917
Models trained or fine-tuned on Marqo/amazon-products-eval
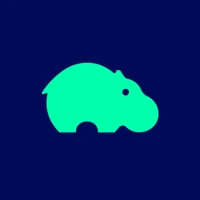