-
PyTorch FSDP: Experiences on Scaling Fully Sharded Data Parallel
Paper • 2304.11277 • Published • 1 -
Megatron-LM: Training Multi-Billion Parameter Language Models Using Model Parallelism
Paper • 1909.08053 • Published • 2 -
Reducing Activation Recomputation in Large Transformer Models
Paper • 2205.05198 • Published -
GPipe: Efficient Training of Giant Neural Networks using Pipeline Parallelism
Paper • 1811.06965 • Published
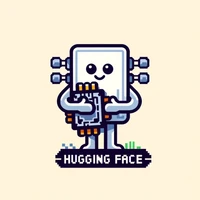
Hugging Face Machine Learning Optimization
AI & ML interests
None defined yet.
Hugging Face Machine Learning Optimizations Team
About Hugging Face's mission
Our mission is to democratize good machine learning.
We want to build the platform for AI builder empowering all the communities towards building collaborative technologies.
Hugging Face is a decentralized, highly impact-oriented, autonomous-driven company.
What does it mean to be part of the Machine Learning Optimization Team at Hugging Face?
Being part of the Machine Learning Optimization Team usually involves new hire to jump into a program with one (or multiple) partner(s) as its main project, supporting Hugging Face overall monetization strategy.
There is no real definition of what projects look like, every partner have different maturity, targets and scopes. We kind of surf over what we observe from a community and Hugging Face products usages to drive the features development with our partners.
While most of the work will usually happen for a partner, we also encourage members of the team to have some time to work on personal project they think would be relevant towards driving more revenues for Hugging Face.
Last but not least, while belonging to the monetization side of the company, we are very central and open-source builders. There are many opportunities to collaborate with other teams and projects from OSS / Community, the Hugging Face Hub and also the Infrastructure...
References
Looking for some real use-cases of what we are diving for Hugging Face? Here is a non-exhausitive list of projects/achievements/sprints we did in the past:
- Hugging Face on AMD Instinct MI300 GPU
- Hugging Face Text Generation Inference available for AWS Inferentia2
- Building Cost-Efficient Enterprise RAG applications with Intel Gaudi 2 and Intel Xeon
- Fast Inference on Large Language Models: BLOOMZ on Habana Gaudi2 Accelerator
- Scaling up BERT-like model Inference on modern CPU
Collections
2
-
AWQ: Activation-aware Weight Quantization for LLM Compression and Acceleration
Paper • 2306.00978 • Published • 9 -
GPTQ: Accurate Post-Training Quantization for Generative Pre-trained Transformers
Paper • 2210.17323 • Published • 8 -
The Era of 1-bit LLMs: All Large Language Models are in 1.58 Bits
Paper • 2402.17764 • Published • 608