Moritz Laurer
AI & ML interests
Recent Activity
Articles
Organizations
MoritzLaurer's activity
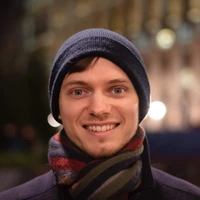
π The paper introduces rStar-Math, which claims to rival OpenAI o1's math reasoning capabilities by integrating Monte Carlo Tree Search (MCTS) with step-by-step verified reasoning trajectories.
π€ A Process Preference Model (PPM) enables fine-grained evaluation of intermediate steps, improving training data quality.
π§ͺ The system underwent four rounds of self-evolution, progressively refining both the policy and reward models to tackle Olympiad-level math problemsβwithout GPT-4-based data distillation.
πΎ While we wait for the release of code and datasets, you can already download the prompts they used from the HF Hub!
Details and links here π
Prompt-templates docs: https://moritzlaurer.github.io/prompt_templates/
Templates on the hub: MoritzLaurer/rstar-math-prompts
Prompt-templates collection: MoritzLaurer/prompt-templates-6776aa0b0b8a923957920bb4
Paper: https://arxiv.org/pdf/2501.04519
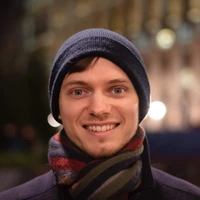
If your data exceeds quantity & quality thresholds and is approved into the next hexgrad/Kokoro-82M training mix, and you permissively DM me the data under an effective Apache license, then I will DM back the corresponding voicepacks for YOUR data if/when the next Apache-licensed Kokoro base model drops.
What does this mean? If you've been calling closed-source TTS or audio API endpoints to:
- Build voice agents
- Make long-form audio, like audiobooks or podcasts
- Handle customer support, etc
Then YOU can contribute to the training mix and get useful artifacts in return. β€οΈ
More details at hexgrad/Kokoro-82M#21
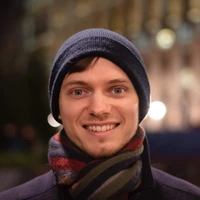
π The paper introduces the FACTS Grounding benchmark for evaluating the factuality of LLM outputs.
π€ Fact-checking is automated by an ensemble of LLM judges that verify if a response is fully grounded in a factual reference document.
π§ͺ The authors tested different prompt templates on held-out data to ensure their generalization.
π It's highly educational to read these templates to learn how frontier labs design prompts and understand their limitations.
πΎ You can now download and reuse these prompt templates via the prompt-templates library!
π The library simplifies sharing prompt templates on the HF hub or locally via standardized YAML files. Letβs make LLM work more transparent and reproducible by sharing more templates like this!
Links π
- prompt-templates docs: https://moritzlaurer.github.io/prompt_templates/
- all templates on the HF Hub: MoritzLaurer/facts-grounding-prompts
- FACTS paper: https://storage.googleapis.com/deepmind-media/FACTS/FACTS_grounding_paper.pdf
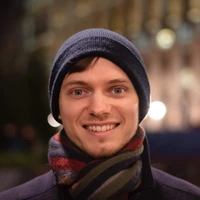
Let's unwrap! merve/jan-10-releases-677fe34177759de0edfc9714
Multimodal πΌοΈ
> ByteDance released SA2VA: a family of vision LMs that can take image, video, text and visual prompts
> moondream2 is out with new capabilities like outputting structured data and gaze detection!
> Dataset: Alibaba DAMO lab released multimodal textbook β 22k hours worth of samples from instruction videos π€―
> Dataset: SciCap captioning on scientific documents benchmark dataset is released along with the challenge!
LLMs π¬
> Microsoft released Phi-4, sota open-source 14B language model π₯
> Dolphin is back with Dolphin 3.0 Llama 3.1 8B π¬π¬
> Prime-RL released Eurus-2-7B-PRIME a new language model trained using PRIME alignment
> SmallThinker-3B is a new small reasoning LM based on Owen2.5-3B-Instruct π
> Dataset: QWQ-LONGCOT-500K is the dataset used to train SmallThinker, generated using QwQ-32B-preview π
> Dataset: @cfahlgren1 released React Code Instructions: a dataset of code instruction-code pairs π
> Dataset: Qwen team is on the roll, they just released CodeElo, a dataset of code preferences π©π»βπ»
Embeddings π
> @MoritzLaurer released zero-shot version of ModernBERT large π
> KaLM is a new family of performant multilingual embedding models with MIT license built using Qwen2-0.5B
Image/Video Generation β―οΈ
> NVIDIA released Cosmos, a new family of diffusion/autoregressive World Foundation Models generating worlds from images, videos and texts π₯
> Adobe released TransPixar: a new text-to-video model that can generate assets with transparent backgrounds (a first!)
> Dataset: fal released cosmos-openvid-1m Cosmos-tokenized OpenVid-1M with samples from OpenVid-1M
Others
> Prior Labs released TabPFNv2, the best tabular transformer is out for classification and regression
> Metagene-1 is a new RNA language model that can be used for pathogen detection, zero-shot embedding and genome understanding
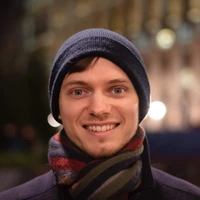
π§ Process reward trainer: Enables training of Process-supervised Reward Models (PRMs), which reward the quality of intermediate steps, promoting structured reasoning. Perfect for tasks like stepwise reasoning.
π Model merging: A new callback leverages mergekit to merge models during training, improving performance by blending reference and policy models - optionally pushing merged models to the Hugging Face Hub.
π οΈ Tool call support: TRL preprocessing now supports tool integration, laying the groundwork for agent fine-tuning with examples like dynamic temperature fetching in prompts.
βοΈ Mixture of judges: The new AllTrueJudge combines decisions from multiple binary judges for more nuanced evaluation.
Read the release notes and other resources here π
Release: https://github.com/huggingface/trl/releases/tag/v0.13.0
Mergekit: https://github.com/arcee-ai/mergekit
Mixture of judges paper: The Perfect Blend: Redefining RLHF with Mixture of Judges (2409.20370)
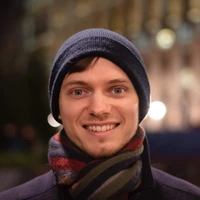
Langfuse brings end-to-end observability and tooling to accelerate your dev workflow from experiments through production
Now available as a Docker Space directly on the HF Hub! π€
π Trace everything: monitor LLM calls, retrieval, and agent actions with popular frameworks
1β£ One-click deployment: on Spaces with persistent storage and integrated OAuth
π Simple Prompt Management: Version, edit, and update without redeployment
β Intuitive Evals: Collect user feedback, run model/prompt evaluations, and improve quality
π Dataset Creation: Build datasets directly from production data to enhance future performance
Kudos to the Langfuse team for this collab and the awesome, open-first product theyβre building! π @marcklingen @Clemo @MJannik
π Space: langfuse/langfuse-template-space
π Docs: https://huggingface.co./docs/hub/spaces-sdks-docker-langfuse
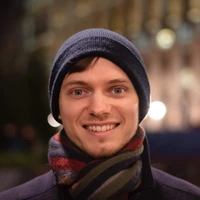
- ChatGPT Pro costs $200/month ($2,400/year) and is still unprofitable for OpenAI due to higher-than-expected usage.
- OpenAI reportedly expected losses of about $5 billion on revenue of $3.7 billion last year, with ChatGPT alone once costing an estimated $700,000 per day to operate. πΈπ₯
- They build strong models and do great research. Whether this business model will work in the long run is one of the biggest questions in the AI economy today.
Source with the numbers π
https://techcrunch.com/2025/01/05/openai-is-losing-money-on-its-pricey-chatgpt-pro-plan-ceo-sam-altman-says/
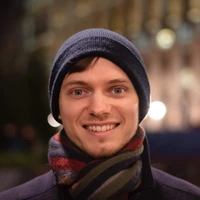
- β‘ Speed & efficiency: It's multiple times faster and uses significantly less memory than DeBERTav3. You can use larger batch sizes and enabling bf16 (instead of fp16) gave me a ~2x speed boost as well
- π Performance tradeoff: It performs slightly worse than DeBERTav3 on average across my zeroshot classification task collection
- π§ Use cases: I recommend using it for scenarios requiring speed and a larger context window (8k).
- π‘ Whatβs next? Iβm preparing a newer version trained on better + longer synthetic data to fully leverage the 8k context window and improve upon the training mix of my older zeroshot-v2.0 models. I also hope that there will be a multilingual variant in the future.
Great work by https://huggingface.co./answerdotai !
If youβre looking for a high-speed zeroshot classifier, give it a try!
π Resources below: π
Base model: MoritzLaurer/ModernBERT-base-zeroshot-v2.0
Large model: MoritzLaurer/ModernBERT-large-zeroshot-v2.0
Updated zeroshot collection: MoritzLaurer/zeroshot-classifiers-6548b4ff407bb19ff5c3ad6f
ModernBERT collection with paper: answerdotai/modernbert-67627ad707a4acbf33c41deb
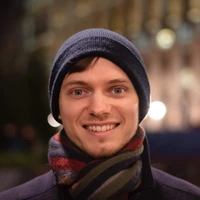
I'm experiencing the same, will release a 0-shot model based on ModernBERT soon
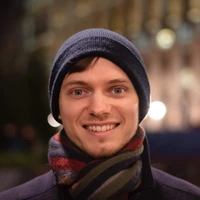
This will probably be the basis for many future SOTA encoders! And I can finally stop using DeBERTav3 from 2021 :D
Congrats @answerdotai , @LightOnIO and collaborators like @tomaarsen !
Paper and models here πhttps://huggingface.co./collections/answerdotai/modernbert-67627ad707a4acbf33c41deb
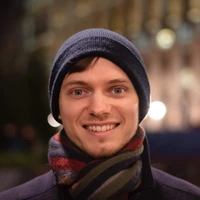
Hey
@borowis
, I don't think there is a plan to add embedding models to the NIM API. Embedding models are quite small which makes them easier to run on accessible hardware (vs. the H100 GPUs running the large LLMs on the NIM API). I'd recommend using a cheap GPU (or even a CPU) via the HF dedicated endpoints for deploying embedding models: https://huggingface.co./inference-endpoints/dedicated And you can use the autoscaling/scale-to-zero feature to avoid unnecessary costs
(The smaller BGE models from the MTEB leaderboard are always a good place to start)
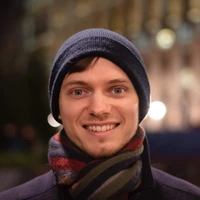
huggingface/open-source-ai-year-in-review-2024
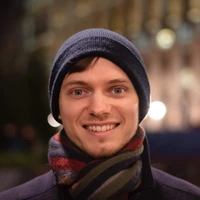
pip install prompt-templates
. Motivation: The community currently shares prompt templates in a wide variety of formats: in datasets, in model cards, as strings in .py files, as .txt/.yaml/.json/.jinja2 files etc. This makes sharing and working with prompt templates unnecessarily complicated.
Prompt templates are currently the main hyperparameter that people tune when building complex LLM systems or agents. If we don't have a common standard for sharing them, we cannot systematically test and improve our systems. After comparing different community approaches, I think that working with modular .yaml or .json files is the best approach.
The
prompt-templates
library : - proposes a standard for sharing prompts (entirely locally or on the HF hub)
- provides some utilities that are interoperable with the broader ecosystem
Try it:
# !pip install prompt-templates
from prompt_templates import PromptTemplateLoader
prompt_template = PromptTemplateLoader.from_hub(repo_id="MoritzLaurer/closed_system_prompts", filename="claude-3-5-artifacts-leak-210624.yaml")
The library is in early stages, feedback is welcome!
More details in the docs: https://github.com/MoritzLaurer/prompt_templates/
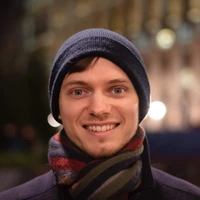
TL;DR:
- public storage is free, and (unless blatant abuse) unlimited. We do ask that you consider upgrading to PRO and/or Enterprise Hub if possible
- private storage is paid above a significant free tier (1TB if you have a paid account, 100GB otherwise)
docs: https://huggingface.co./docs/hub/storage-limits
We optimize our infrastructure continuously to scale our storage for the coming years of growth in Machine learning, to the benefit of the community π₯
cc: @reach-vb @pierric @victor and the HF team
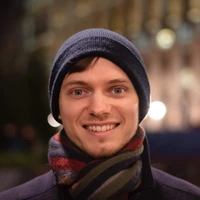
The reference names are always in this collection for the NIM API: https://huggingface.co./collections/nvidia/nim-serverless-inference-api-66a3c6fcdcb5bbc6e975b508
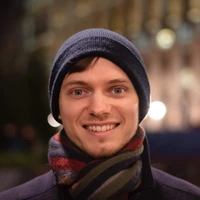
It works for me with this code actually. The small change I made is a minimal change in the model identifier (removing the "meta"). The issue is that Meta renamed their models on HF recently.
It works for me with this code. Can you try again with this code?
#!pip install "huggingface_hub>=0.25.0"
from huggingface_hub import InferenceClient
client = InferenceClient(
base_url="https://huggingface.co./api/integrations/dgx/v1",
api_key=os.getenv("HF_TOKEN_ENTERPRISE") # see docs: https://huggingface.co./blog/inference-dgx-cloud#create-a-fine-grained-token
)
output = client.chat.completions.create(
model="meta-llama/Llama-3.1-405B-Instruct-FP8", #"meta-llama/Meta-Llama-3.1-405B-Instruct-FP8",
messages=[
{"role": "system", "content": "You are a helpful assistant."},
{"role": "user", "content": "Count to 10"},
],
max_tokens=1024,
)
print(output)
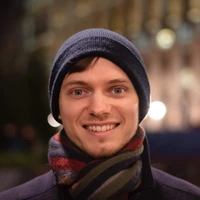
ok, I could reproduce the issue and I get the same error, I'm reporting it to Nvidia, thanks for reporting
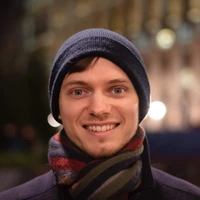
What error do you get for the 405b?
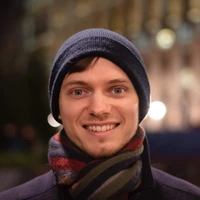
Hey @jmparejaz , did you use a token from an enterprise org as explained here https://huggingface.co./blog/inference-dgx-cloud#create-a-fine-grained-token ?